News
The Threat of Deepfakes: AI and ML in the Fight Against Synthetic Media
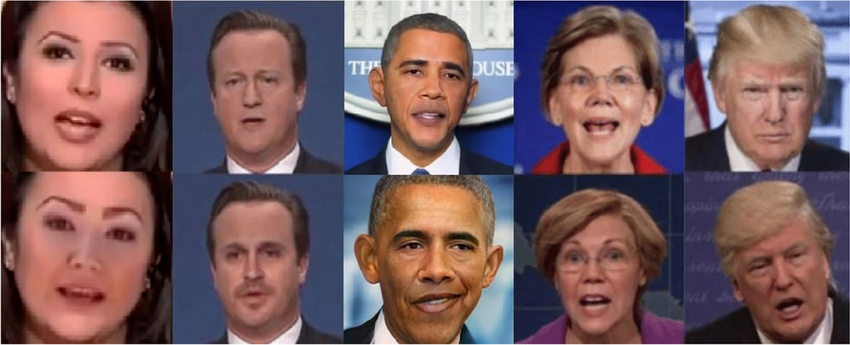
The Threat of Deepfakes: AI and ML in the Fight Against Synthetic Media
Deepfakes, a type of synthetic media produced using artificial intelligence have lately been somewhat well-known due to their incredible realism in audio, video, and picture manipulation. Deepfakes, which are now used for public dishonesty, false information distribution, and reputation damage, have progressed from a benign form of online amusement to a major cause of worry. Their increasing complexity makes it more difficult to separate actual from fake information. To keep people’s trust in news sources in today’s fast-paced, social media-driven media environment, deepfakes must be identified and prevented. Given the prospective disruption of political processes, effects on financial markets, and erosion of public trust brought about by deepfakes, effective detection techniques are important. Artificial intelligence (AI) and machine learning (ML) are used to neutralise this threat.
AI driven systems are advancing in their capacity to detect deepfakes using pattern, anomaly, and deviation analysis of audiovisual data. AI uses deep learning and neural networks to enable the recognition of changing material, therefore protecting digital platforms from the dangers of synthetic content. This article covers the significance of artificial intelligence and machine learning in the battle against deepfakes as well as the continuous developments of these technologies.
The Evolution of Deepfakes
From simple picture editing to complex film and audio creation, deepfakes have developed over time. Though the technique originated in early work on face recognition in the 1990s, the word “deepfake” did not initially come up until 2017. Deepfakes might overcome its initial limitation (that of face-swapping in still images) partly due to developments in machine learning, especially Generative Adversarial Networks (GANs). Using a generator of phoney material and a discriminator, the GANs introduced in 2014 aim to distinguish between real and fake. This competitive process continually improves the quality of contents that are generated. By 2018, deepfake technology had progressed to creating convincing video and audio, sparking both awe and concern.
Today, deepfakes can manipulate facial expressions, lip movements, and voice in real-time, blurring the line between reality and fiction. This technology has found applications in entertainment and education but also poses significant challenges. Privacy concerns arise as anyone’s likeness can be co-opted without consent. Security threats emerge from potential misuse in fraud or disinformation campaigns. Perhaps most critically, deepfakes erode trust in digital media, making it increasingly difficult to discern authentic content from fabrications.
AI and Machine Learning Approaches to Detecting Deepfakes
Detecting deepfakes requires sophisticated AI and machine learning (ML) techniques capable of recognizing subtle anomalies in synthetic content. Deepfakes, generated using techniques like Generative Adversarial Networks (GANs), often contain small discrepancies that are difficult for the human eye to detect but can be identified through AI-powered analysis. AI-based detection techniques leverage vast amounts of data and advanced algorithms to learn patterns that differentiate real media from deepfakes.
- Neural Network: One of the primary AI techniques used is neural networks, specifically deep learning models like Convolutional Neural Networks (CNNs). CNNs are widely employed because they can process visual information, analyze patterns, and detect inconsistencies in images and videos. By training on real and fake datasets, CNNs learn to spot irregularities in the pixel structure or frame transitions that signal manipulation. These networks are capable of detecting the subtle differences between a real human face and a digitally altered one, even if the deepfake is highly realistic.
- Facial Movement Analysis: Real faces move naturally, with specific muscle patterns and micro-expressions that deepfake algorithms struggle to replicate perfectly. AI models analyse these facial dynamics, tracking the synchronization between lip movement and audio, eye blinking rates, or slight shifts in facial muscles to detect discrepancies in manipulated videos.
- Audio Inconsistencies: Deepfake videos often feature mismatches between the audio track and the visual content. For instance, AI can pick up on unnatural speech patterns, irregularities in sound frequency, or mismatches between mouth movements and spoken words. These abnormalities can be flagged by AI systems designed to match audio to visuals.
- Pixel-level Analysis: This is another effective AI-driven approach. Deepfake generators, while sophisticated, tend to leave pixel anomalies, particularly at the boundary of manipulated regions (e.g., around the eyes, mouth, or skin texture). AI can detect these pixel-level irregularities, which are often too subtle for human viewers to notice but are indicative of digital tampering.
- AI-Powered Spatial and Temporal Analysis: This can scrutinize video frames for inconsistencies across time. While an individual frame may appear realistic, examining the sequence of frames often reveals inconsistencies in motion or lighting, which deepfakes fail to maintain consistently. These subtle distortions can signal that a video has been digitally altered.
The Arms Race: Deepfake Creation vs. Detection
The battle between deepfake creators and detectors is a continuous arms race. As AI and machine learning tools improve at detecting synthetic media, deepfake creators respond by refining their techniques, making detection increasingly difficult. This cycle of advancement is driven by the dual capabilities of AI, which plays a key role in both the creation and detection of deepfakes.
On one side, deepfake creators leverage technologies like Generative Adversarial Networks (GANs) to produce increasingly sophisticated synthetic media. GANs consist of two neural networks—the generator, which creates fake content, and the discriminator, which attempts to identify real versus fake content. As the discriminator improves, the generator learns to produce even more realistic deepfakes, creating an ever-evolving challenge for detection systems. This feedback loop enables deepfake creators to generate media that are harder to distinguish from authentic content. On the other side, advancements in AI detection technologies prompt creators to innovate further. For example, when facial movement analysis became a popular detection method, deepfake algorithms improved their ability to replicate natural facial dynamics. Similarly, pixel-level analysis of deepfakes spurred creators to enhance image resolution and reduce detectable inconsistencies. As detection techniques evolve, so do the methods of countering them, resulting in a constant tug-of-war.
AI itself is central to both sides of this arms race. The same machine learning models that power detection systems also underpin deepfake generation tools. This dual role of AI presents a unique challenge—while it helps in defending against synthetic media, it also serves as the foundation for producing increasingly convincing deepfakes. The result is a perpetual cycle of creation and detection, where advances on one side directly fuel innovation on the other. This arms race continues to shape the future of media integrity and security.
Key Technologies in Deepfake Detection
The rapid advancement of deepfakes has prompted the development of sophisticated AI and machine learning technologies to detect synthetic content. These technologies harness the power of neural networks, deep learning models, and advanced forensics techniques to identify even the most subtle manipulations. Here’s a detailed look at some of the top technologies used in deepfake detection.
- Convolutional Neural Networks (CNNs): Convolutional Neural Networks (CNNs) are among the most widely used AI tools in deepfake detection. CNNs excel at processing and analyzing visual data, making them ideal for detecting image and video anomalies. By breaking down visual content into smaller pixel-level units, CNNs can identify inconsistencies that are typically invisible to the human eye. For example, they can detect subtle differences in skin texture, lighting, and facial expressions across frames. Trained on massive datasets of real and fake media, CNNs learn to spot even the slightest signs of tampering. Their ability to handle complex visual data makes them central to the detection of deepfake videos.
- GANs for Detecting Deepfakes: Generative Adversarial Networks (GANs), the very technology used to create deepfakes, are also employed in detecting them. GANs consist of two neural networks: a generator that creates fake content and a discriminator that tries to distinguish between real and fake. In detection, GANs are used to reverse-engineer deepfake generation processes by analysing and comparing real content with synthetically produced media. Detection-focused GANs excel at identifying unusual artifacts in deepfake videos, such as inconsistencies in lighting, facial alignment, or audio mismatches.
- Audio-Visual Forensics: Audio-visual forensics integrates AI-driven techniques to analyse both the video and audio components of media. Deepfakes often struggle to perfectly sync voice and facial movements, creating detectable discrepancies. By analysing the synchronization between lip movement and speech, AI algorithms can detect subtle differences that suggest manipulation. Additionally, deepfakes tend to introduce audio artifacts, such as unnatural pauses or pitch irregularities, which can be flagged by forensic tools. This method is especially useful for catching deepfake videos where the speaker’s words and facial movements don’t align naturally.
- Real-World Applications: AI-based deepfake detection technologies have found crucial applications in various fields. In media, news organizations are employing these tools to verify the authenticity of video content before broadcasting. Security agencies use AI to detect deepfakes in surveillance footage or to prevent the spread of disinformation during elections. In law enforcement, deepfake detection helps combat criminal activities like fraud or impersonation by identifying doctored evidence. Social media platforms are increasingly deploying AI-powered detection tools to remove manipulated content, safeguarding user trust.
Challenges in Deepfake Detection
Despite significant advancements, deepfake detection technologies face several challenges that limit their effectiveness. As deepfake creation methods become more sophisticated, the limitations of current AI and machine learning models are increasingly exposed.
- Limitations of AI Models: One major challenge is the inability of AI models to keep pace with the rapid evolution of deepfake techniques. Deepfake generation tools, especially those based on Generative Adversarial Networks (GANs), are constantly improving, making it harder for existing detection models to identify fakes. Additionally, detection tools often rely on massive datasets for training, and deepfake creators can exploit unseen techniques that the AI hasn’t been trained to detect. This means that newer, more advanced deepfakes may bypass even the most advanced detection algorithms.
- Ethical Concerns and Bias: AI detection systems are not immune to biases. Detection algorithms may perform unevenly across different demographics, such as race, gender, or age, leading to false positives or negatives. For instance, facial recognition and detection models have historically struggled with people of colour due to unbalanced training data, which raises concerns about fairness and inclusivity. Ethical questions also arise when it comes to privacy, as detecting deepfakes may require intrusive data collection, such as facial scans or personal audio recordings, which could infringe on individual rights.
- Accessibility and Open-Source Tools: Many advanced deepfake detection tools are developed by large corporations or government agencies, limiting public access. The lack of open-source detection software means that smaller organizations, independent media outlets, and the general public have fewer resources to detect deepfakes. This disparity in access puts underfunded groups at a disadvantage when combating misinformation. The need for more accessible and open-source tools is crucial in ensuring that everyone can participate in the fight against deepfakes and safeguard the integrity of information.
Future Trends: What Lies Ahead?
The future of deepfake detection is set to be shaped by emerging technologies and innovative approaches aimed at staying ahead of increasingly sophisticated synthetic media. As deepfakes evolve, more accurate and reliable detection tools are needed to safeguard the integrity of digital content. Here are some key trends that are likely to shape the future of deepfake detection.
- Advanced AI Tools: One of the most promising trends is the development of more sophisticated AI tools, such as self-supervised learning and transformer models. Unlike traditional deep learning models that require massive datasets, self-supervised models can learn from smaller data samples, making them more adaptable to new and evolving deepfake techniques. Transformer models, which have revolutionized natural language processing, are being adapted to analyse and cross-verify both visual and audio data, improving detection accuracy. These advanced tools will enhance AI’s ability to identify subtle anomalies in deepfakes.
- Blockchain for Decentralized Verification: Blockchain technology offers a novel solution to the deepfake problem through decentralized media verification. By creating immutable records of media content at the point of creation, blockchain can verify the authenticity of images, videos, and audio files as they circulate online. Any alterations to the original content can be detected through the blockchain ledger, ensuring transparency and accountability. This decentralized approach empowers content creators and consumers to verify the integrity of digital media without relying on centralized platforms.
- AI-Based Content Verification: The future will likely see the integration of AI-based content verification systems across social media platforms, news organizations, and security agencies. These systems could operate in real-time, flagging potential deepfakes as they are uploaded or shared. Combined with technologies like digital watermarking, which embeds hidden, tamper-proof identifiers in media, AI-based systems will offer an automated, scalable solution to deepfake detection.
Conclusion
The ongoing battle against deepfakes highlights the crucial role that AI and machine learning play in preserving the integrity of digital media. Through advanced techniques like CNN, GANs, and audio-visual forensics, these technologies enable the detection of subtle manipulations in synthetic media, helping to safeguard trust in what we consume online. However, the continuous arms race between deepfake creators and detectors underscores the need for ongoing innovation. The continued development of AI-driven detection tools is vital to staying ahead of increasingly sophisticated deepfakes. As the technology evolves, so too must our defences. Ensuring the authenticity of digital content is not just a technical challenge but a societal imperative to protect individuals, institutions, and the broader public from the harmful impacts of misinformation and deception in an ever-expanding digital landscape.
Authors Name: Ahmed Olabisi Olajide (Co-founder Eybrids)
LinkedIn: Olabisi Olajide | LinkedIn
Headlines
Akwa Ibom APC Gears Up to Receive President Tinubu as Governor Umo Eno Joins Party
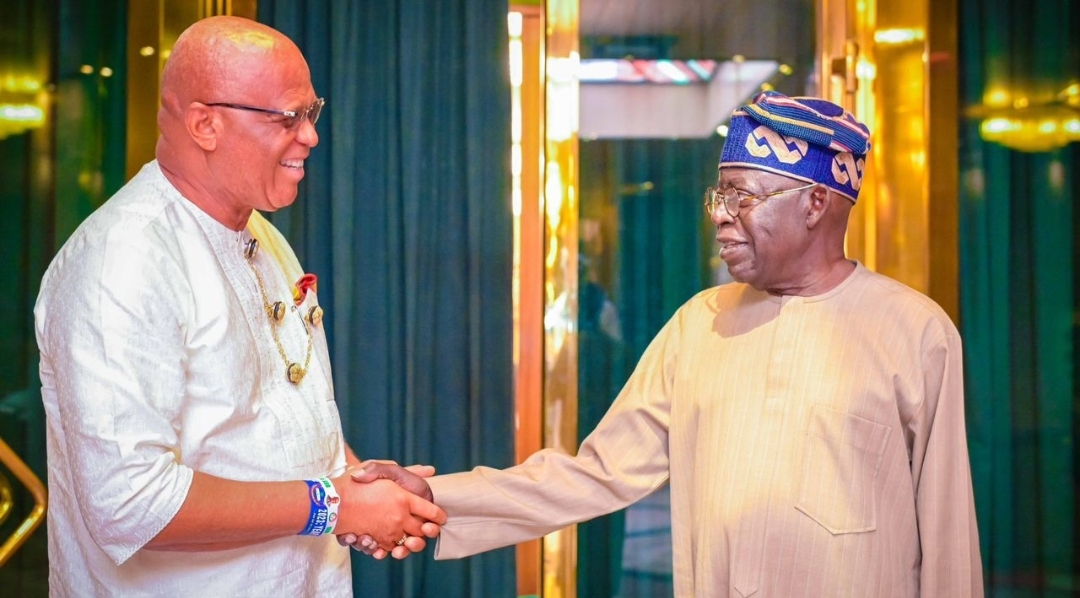
The All Progressives Congress (APC) in Akwa Ibom State has announced its readiness to host President Bola Ahmed Tinubu and other top party leaders as it officially welcomes Governor Umo Eno into its ranks.
Speaking at a press briefing in Uyo on Friday, APC chieftain and former presidential aide, Senator Ita Enang, disclosed that the party was fully prepared to receive the president, Vice President Kashim Shettima, APC National Chairman, and governors elected on the party’s platform for the historic reception.
Governor Umo Eno had on June 6 formally defected from the Peoples Democratic Party (PDP) to the APC, in a move widely described as a political game-changer in Akwa Ibom.
Enang, a former Special Assistant to the President on National Assembly and Niger Delta Affairs, said the governor’s defection had effectively aligned the state with the central government.
He assured Governor Eno and his supporters that the APC would embrace them wholeheartedly and honour all agreements reached.
“As progressives, we shall work with the governor and his supporters to ensure that they fit into the party without hitches,” Enang stated. “We will also work with them to align programmes of the state government with the ideals and manifesto of the party.”
He further described the governor’s move as a “merger” that promises significant benefits for Akwa Ibom and its citizens.
The planned reception is expected to mark a major political event in the state, signaling a realignment of forces ahead of future elections.
Headlines
Ekiti Launches Aggressive Anti-Flood Campaign, Dredges Ofigba River
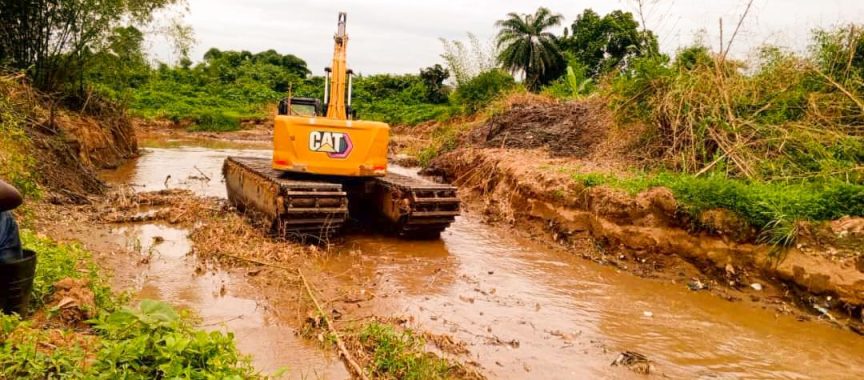
The Ekiti State Government has intensified efforts to prevent flooding across the state with the launch of a comprehensive dredging campaign, targeting critical waterways in both rural and urban areas.
Chairman of the Ekiti State Environmental Protection Agency (EKSEPA), Chief Bamitale Oguntoyinbo, disclosed this on Friday during an inspection visit to the ongoing dredging project at the Ofigba River in Ise-Ekiti.
Oguntoyinbo, who was accompanied by EKSEPA board members, said the visit was to assess the progress of work being carried out to mitigate flood risks in the community. He expressed satisfaction with the pace and quality of the dredging work.
“I and other board members of EKSEPA are delighted with the level of job done by the site engineer because he is actually working with the directives of three-kilometer dredging of waterways,” he said.
According to him, the dredging commenced on June 4, and so far, 1.8 kilometers of the river have been successfully cleared.
He applauded Governor Biodun Oyebanji for prioritizing the safety and welfare of residents by initiating the state-wide anti-flooding campaign.
“I want to commend our amiable governor, Mr. Biodun Oyebanji, for embarking on zero tolerance campaign against flooding in every community and town in Ekiti,” Oguntoyinbo stated.
He also praised the General Manager of EKSEPA, Mr. Olukayode Adunmo, for his commitment to the project’s supervision and success.
In his remarks, Adunmo emphasized the urgent need to clear waterways choked by refuse, which impede water flow and contribute to flooding during the rainy season.
“Dredging of Ofigba River in Ise-Ekiti in Ise/Orun Local Government Area is necessary because some of the waterways have been blocked by refuse,” he explained. “There is the need for us to remove every blockage to enhance free flow of water and avert flooding during heavy rainfall.”
Adunmo also commended Governor Oyebanji for taking proactive steps to protect lives and properties across the state.
Residents of Ise-Ekiti have welcomed the government’s intervention. Chief Godwin Ojo, a community leader, expressed gratitude to the governor for his timely action.
“We thank the governor for the move to avert flooding in our community,” Ojo said. “May God grant him more wisdom to pilot the affairs of the state to an enviable height.”
The dredging campaign forms part of the Oyebanji administration’s broader commitment to environmental safety and disaster prevention.
Developmental
Tinubu to visit Kaduna Thursday to inaugurate key projects
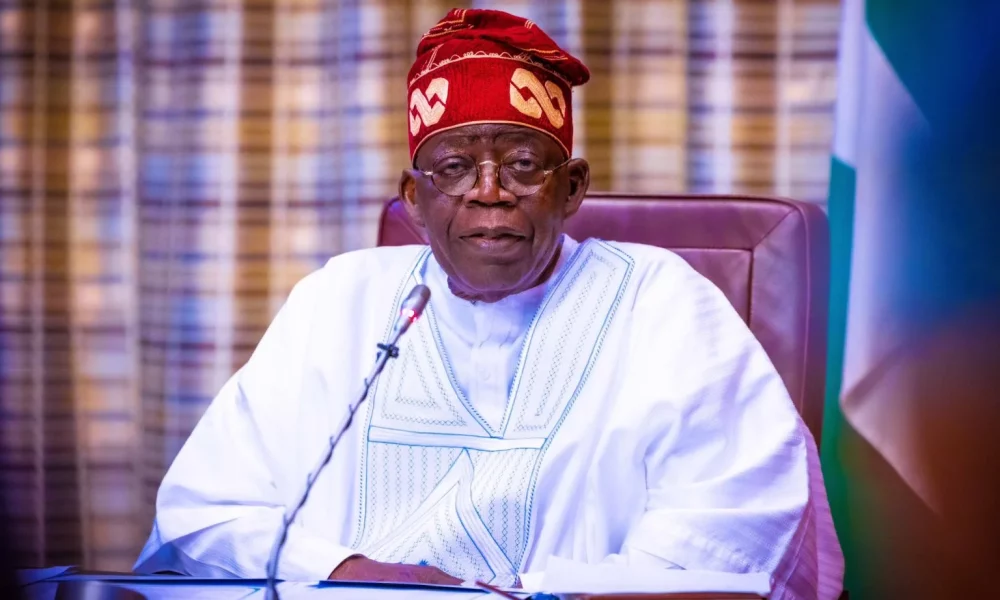
President Bola Tinubu is expected in Kaduna State Today Thursday for the inauguration of several key developmental projects executed by the administration of Gov. Uba Sani.
The News Agency of Nigeria (NAN) reports that the visit forms part of activities marking Sani’s two years in office.
The projects lined up for inauguration include the 300-bed Specialist Hospital in Millennium City, Kaduna, built by the state government to bolster the provision of healthcare services.
Tinubu will also inaugurate the Institute of Vocational Training and Skills Development in Rigachikun, road projects in Soba, and Samaru Kataf LGA’S as well as the 24-kilometre Kafanchan Township Road.
Others are the Tudun Biri Road, the 22km road linking Kauru and Kubau LGAs as well as the Vocational and Skills Training Centre in Tudun Biri.
Tinubu is also expected to unveil 100 Compressed Natural Gas (CNG) buses, as part of efforts to modernise the state’s public transportation system.
The projects are part of the administration’s focus on infrastructurde evelopment, healthcare delivery, youths empowerment, and economic growth.
The state government described the visit as a significant moment for the people of Kaduna and an opportunity to showcase ongoing efforts to transform the state through impactful governance.
Sani, who marked his second year in office this month, has prioritised human capital development, rural infrastructure, and jobs creation since taking office in 2023.
Tinubu’s visit to Kaduna State was rescheduled from Wednesday to Thursday.
He was initially supposed to visit Kaduna on Wednesday, but due to the recent attacks in Benue, he shifted his trip.
The president visited Benue on Wednesday to commiserate with the victims of the recent attacks and assess the humanitarian crisis.
During his visit to Benue, Tinubu met with stakeholders, including traditional rulers, political and community leaders, and youth groups, to seek lasting solutions to the hostilities.
He also condemned the ongoing violence and called on the residents to embrace peace and mutual understanding.
NAN recalls that the Benue Government had declared a work-free day for Tinubu’s visit, urging the residents to turn out in large numbers to welcome him.
-
Headlines3 years ago
Facebook, Instagram Temporarily Allow Posts on Ukraine War Calling for Violence Against Invading Russians or Putin’s Death
-
Headlines3 years ago
Nigeria, Other West African Countries Facing Worst Food Crisis in 10 Years, Aid Groups Say
-
Foreign3 years ago
New York Consulate installs machines for 10-year passport
-
News10 months ago
Zero Trust Architecture in a Remote World: Securing the New Normal
-
Entertainment3 years ago
Phyna emerges winner of Big Brother Naija Season 7
-
Headlines1 year ago
Nigeria Customs modernisation project to check extortion of traders
-
Economy1 year ago
We generated N30.2 bn revenue in three months – Kano NCS Comptroller
-
Headlines1 year ago
Philippines’ Vice President Sara Duterte resigns from Cabinet