Technology
The Threat of Deepfakes: AI and ML in the Fight Against Synthetic Media
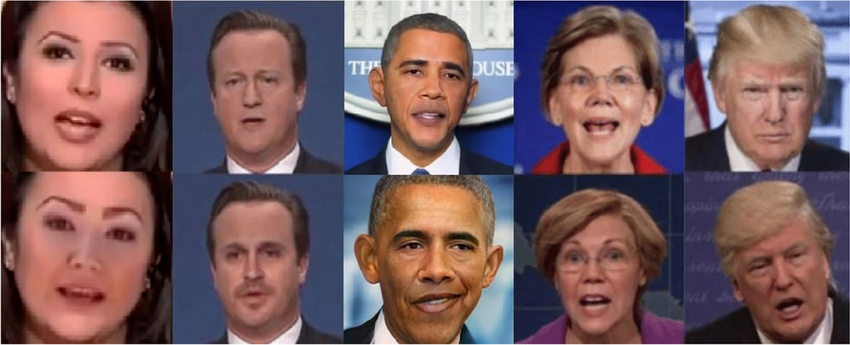
The Threat of Deepfakes: AI and ML in the Fight Against Synthetic Media
Deepfakes, a type of synthetic media produced using artificial intelligence have lately been somewhat well-known due to their incredible realism in audio, video, and picture manipulation. Deepfakes, which are now used for public dishonesty, false information distribution, and reputation damage, have progressed from a benign form of online amusement to a major cause of worry. Their increasing complexity makes it more difficult to separate actual from fake information. To keep people’s trust in news sources in today’s fast-paced, social media-driven media environment, deepfakes must be identified and prevented. Given the prospective disruption of political processes, effects on financial markets, and erosion of public trust brought about by deepfakes, effective detection techniques are important. Artificial intelligence (AI) and machine learning (ML) are used to neutralise this threat.
AI driven systems are advancing in their capacity to detect deepfakes using pattern, anomaly, and deviation analysis of audiovisual data. AI uses deep learning and neural networks to enable the recognition of changing material, therefore protecting digital platforms from the dangers of synthetic content. This article covers the significance of artificial intelligence and machine learning in the battle against deepfakes as well as the continuous developments of these technologies.
The Evolution of Deepfakes
From simple picture editing to complex film and audio creation, deepfakes have developed over time. Though the technique originated in early work on face recognition in the 1990s, the word “deepfake” did not initially come up until 2017. Deepfakes might overcome its initial limitation (that of face-swapping in still images) partly due to developments in machine learning, especially Generative Adversarial Networks (GANs). Using a generator of phoney material and a discriminator, the GANs introduced in 2014 aim to distinguish between real and fake. This competitive process continually improves the quality of contents that are generated. By 2018, deepfake technology had progressed to creating convincing video and audio, sparking both awe and concern.
Today, deepfakes can manipulate facial expressions, lip movements, and voice in real-time, blurring the line between reality and fiction. This technology has found applications in entertainment and education but also poses significant challenges. Privacy concerns arise as anyone’s likeness can be co-opted without consent. Security threats emerge from potential misuse in fraud or disinformation campaigns. Perhaps most critically, deepfakes erode trust in digital media, making it increasingly difficult to discern authentic content from fabrications.
AI and Machine Learning Approaches to Detecting Deepfakes
Detecting deepfakes requires sophisticated AI and machine learning (ML) techniques capable of recognizing subtle anomalies in synthetic content. Deepfakes, generated using techniques like Generative Adversarial Networks (GANs), often contain small discrepancies that are difficult for the human eye to detect but can be identified through AI-powered analysis. AI-based detection techniques leverage vast amounts of data and advanced algorithms to learn patterns that differentiate real media from deepfakes.
- Neural Network: One of the primary AI techniques used is neural networks, specifically deep learning models like Convolutional Neural Networks (CNNs). CNNs are widely employed because they can process visual information, analyze patterns, and detect inconsistencies in images and videos. By training on real and fake datasets, CNNs learn to spot irregularities in the pixel structure or frame transitions that signal manipulation. These networks are capable of detecting the subtle differences between a real human face and a digitally altered one, even if the deepfake is highly realistic.
- Facial Movement Analysis: Real faces move naturally, with specific muscle patterns and micro-expressions that deepfake algorithms struggle to replicate perfectly. AI models analyse these facial dynamics, tracking the synchronization between lip movement and audio, eye blinking rates, or slight shifts in facial muscles to detect discrepancies in manipulated videos.
- Audio Inconsistencies: Deepfake videos often feature mismatches between the audio track and the visual content. For instance, AI can pick up on unnatural speech patterns, irregularities in sound frequency, or mismatches between mouth movements and spoken words. These abnormalities can be flagged by AI systems designed to match audio to visuals.
- Pixel-level Analysis: This is another effective AI-driven approach. Deepfake generators, while sophisticated, tend to leave pixel anomalies, particularly at the boundary of manipulated regions (e.g., around the eyes, mouth, or skin texture). AI can detect these pixel-level irregularities, which are often too subtle for human viewers to notice but are indicative of digital tampering.
- AI-Powered Spatial and Temporal Analysis: This can scrutinize video frames for inconsistencies across time. While an individual frame may appear realistic, examining the sequence of frames often reveals inconsistencies in motion or lighting, which deepfakes fail to maintain consistently. These subtle distortions can signal that a video has been digitally altered.
The Arms Race: Deepfake Creation vs. Detection
The battle between deepfake creators and detectors is a continuous arms race. As AI and machine learning tools improve at detecting synthetic media, deepfake creators respond by refining their techniques, making detection increasingly difficult. This cycle of advancement is driven by the dual capabilities of AI, which plays a key role in both the creation and detection of deepfakes.
On one side, deepfake creators leverage technologies like Generative Adversarial Networks (GANs) to produce increasingly sophisticated synthetic media. GANs consist of two neural networks—the generator, which creates fake content, and the discriminator, which attempts to identify real versus fake content. As the discriminator improves, the generator learns to produce even more realistic deepfakes, creating an ever-evolving challenge for detection systems. This feedback loop enables deepfake creators to generate media that are harder to distinguish from authentic content. On the other side, advancements in AI detection technologies prompt creators to innovate further. For example, when facial movement analysis became a popular detection method, deepfake algorithms improved their ability to replicate natural facial dynamics. Similarly, pixel-level analysis of deepfakes spurred creators to enhance image resolution and reduce detectable inconsistencies. As detection techniques evolve, so do the methods of countering them, resulting in a constant tug-of-war.
AI itself is central to both sides of this arms race. The same machine learning models that power detection systems also underpin deepfake generation tools. This dual role of AI presents a unique challenge—while it helps in defending against synthetic media, it also serves as the foundation for producing increasingly convincing deepfakes. The result is a perpetual cycle of creation and detection, where advances on one side directly fuel innovation on the other. This arms race continues to shape the future of media integrity and security.
Key Technologies in Deepfake Detection
The rapid advancement of deepfakes has prompted the development of sophisticated AI and machine learning technologies to detect synthetic content. These technologies harness the power of neural networks, deep learning models, and advanced forensics techniques to identify even the most subtle manipulations. Here’s a detailed look at some of the top technologies used in deepfake detection.
- Convolutional Neural Networks (CNNs): Convolutional Neural Networks (CNNs) are among the most widely used AI tools in deepfake detection. CNNs excel at processing and analyzing visual data, making them ideal for detecting image and video anomalies. By breaking down visual content into smaller pixel-level units, CNNs can identify inconsistencies that are typically invisible to the human eye. For example, they can detect subtle differences in skin texture, lighting, and facial expressions across frames. Trained on massive datasets of real and fake media, CNNs learn to spot even the slightest signs of tampering. Their ability to handle complex visual data makes them central to the detection of deepfake videos.
- GANs for Detecting Deepfakes: Generative Adversarial Networks (GANs), the very technology used to create deepfakes, are also employed in detecting them. GANs consist of two neural networks: a generator that creates fake content and a discriminator that tries to distinguish between real and fake. In detection, GANs are used to reverse-engineer deepfake generation processes by analysing and comparing real content with synthetically produced media. Detection-focused GANs excel at identifying unusual artifacts in deepfake videos, such as inconsistencies in lighting, facial alignment, or audio mismatches.
- Audio-Visual Forensics: Audio-visual forensics integrates AI-driven techniques to analyse both the video and audio components of media. Deepfakes often struggle to perfectly sync voice and facial movements, creating detectable discrepancies. By analysing the synchronization between lip movement and speech, AI algorithms can detect subtle differences that suggest manipulation. Additionally, deepfakes tend to introduce audio artifacts, such as unnatural pauses or pitch irregularities, which can be flagged by forensic tools. This method is especially useful for catching deepfake videos where the speaker’s words and facial movements don’t align naturally.
- Real-World Applications: AI-based deepfake detection technologies have found crucial applications in various fields. In media, news organizations are employing these tools to verify the authenticity of video content before broadcasting. Security agencies use AI to detect deepfakes in surveillance footage or to prevent the spread of disinformation during elections. In law enforcement, deepfake detection helps combat criminal activities like fraud or impersonation by identifying doctored evidence. Social media platforms are increasingly deploying AI-powered detection tools to remove manipulated content, safeguarding user trust.
Challenges in Deepfake Detection
Despite significant advancements, deepfake detection technologies face several challenges that limit their effectiveness. As deepfake creation methods become more sophisticated, the limitations of current AI and machine learning models are increasingly exposed.
- Limitations of AI Models: One major challenge is the inability of AI models to keep pace with the rapid evolution of deepfake techniques. Deepfake generation tools, especially those based on Generative Adversarial Networks (GANs), are constantly improving, making it harder for existing detection models to identify fakes. Additionally, detection tools often rely on massive datasets for training, and deepfake creators can exploit unseen techniques that the AI hasn’t been trained to detect. This means that newer, more advanced deepfakes may bypass even the most advanced detection algorithms.
- Ethical Concerns and Bias: AI detection systems are not immune to biases. Detection algorithms may perform unevenly across different demographics, such as race, gender, or age, leading to false positives or negatives. For instance, facial recognition and detection models have historically struggled with people of colour due to unbalanced training data, which raises concerns about fairness and inclusivity. Ethical questions also arise when it comes to privacy, as detecting deepfakes may require intrusive data collection, such as facial scans or personal audio recordings, which could infringe on individual rights.
- Accessibility and Open-Source Tools: Many advanced deepfake detection tools are developed by large corporations or government agencies, limiting public access. The lack of open-source detection software means that smaller organizations, independent media outlets, and the general public have fewer resources to detect deepfakes. This disparity in access puts underfunded groups at a disadvantage when combating misinformation. The need for more accessible and open-source tools is crucial in ensuring that everyone can participate in the fight against deepfakes and safeguard the integrity of information.
Future Trends: What Lies Ahead?
The future of deepfake detection is set to be shaped by emerging technologies and innovative approaches aimed at staying ahead of increasingly sophisticated synthetic media. As deepfakes evolve, more accurate and reliable detection tools are needed to safeguard the integrity of digital content. Here are some key trends that are likely to shape the future of deepfake detection.
- Advanced AI Tools: One of the most promising trends is the development of more sophisticated AI tools, such as self-supervised learning and transformer models. Unlike traditional deep learning models that require massive datasets, self-supervised models can learn from smaller data samples, making them more adaptable to new and evolving deepfake techniques. Transformer models, which have revolutionized natural language processing, are being adapted to analyse and cross-verify both visual and audio data, improving detection accuracy. These advanced tools will enhance AI’s ability to identify subtle anomalies in deepfakes.
- Blockchain for Decentralized Verification: Blockchain technology offers a novel solution to the deepfake problem through decentralized media verification. By creating immutable records of media content at the point of creation, blockchain can verify the authenticity of images, videos, and audio files as they circulate online. Any alterations to the original content can be detected through the blockchain ledger, ensuring transparency and accountability. This decentralized approach empowers content creators and consumers to verify the integrity of digital media without relying on centralized platforms.
- AI-Based Content Verification: The future will likely see the integration of AI-based content verification systems across social media platforms, news organizations, and security agencies. These systems could operate in real-time, flagging potential deepfakes as they are uploaded or shared. Combined with technologies like digital watermarking, which embeds hidden, tamper-proof identifiers in media, AI-based systems will offer an automated, scalable solution to deepfake detection.
Conclusion
The ongoing battle against deepfakes highlights the crucial role that AI and machine learning play in preserving the integrity of digital media. Through advanced techniques like CNN, GANs, and audio-visual forensics, these technologies enable the detection of subtle manipulations in synthetic media, helping to safeguard trust in what we consume online. However, the continuous arms race between deepfake creators and detectors underscores the need for ongoing innovation. The continued development of AI-driven detection tools is vital to staying ahead of increasingly sophisticated deepfakes. As the technology evolves, so too must our defences. Ensuring the authenticity of digital content is not just a technical challenge but a societal imperative to protect individuals, institutions, and the broader public from the harmful impacts of misinformation and deception in an ever-expanding digital landscape.
Authors Name: Ahmed Olabisi Olajide (Co-founder Eybrids)
LinkedIn: Olabisi Olajide | LinkedIn
Technology
The Price of Neglect: The Economic Impact of Cyberattacks on Maritime Operations
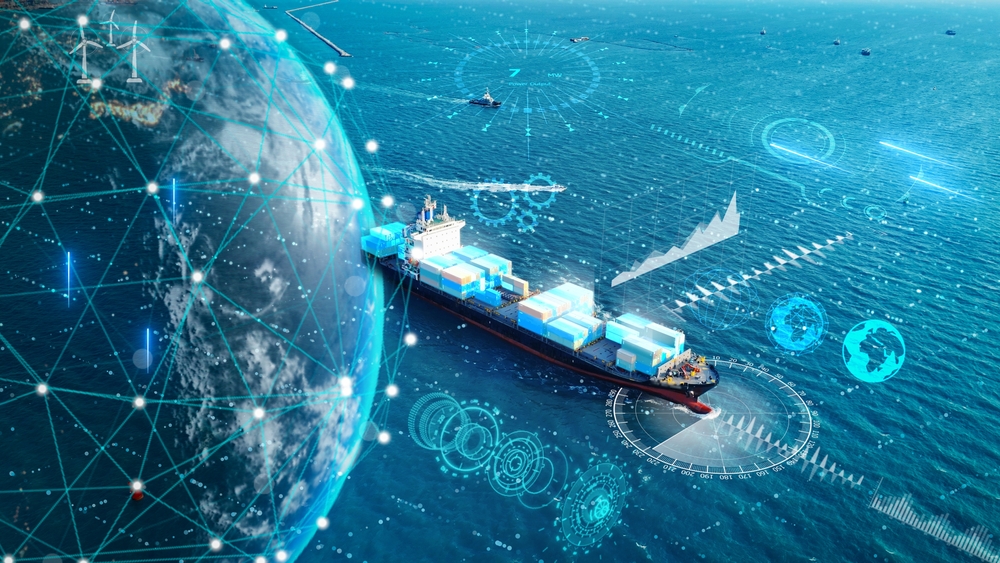
The Price of Neglect: The Economic Impact of Cyberattacks on Maritime Operations
By Abuh Ibrahim Sani
Ports are critical infrastructure to countries economic growth and sustainability. Over 90% of nations around the world depends on importation and exportation of goods. The maritime sector has become an integral part of global trade, connecting markets and facilitating the movement of goods across regions and continents. However, as with other sectors, the growing dependence on digital systems has exposed maritime operations to the growing threat of cyberattack. These attacks have dire economic consequences, as seen in countries like USA, Nigeria, Japan, China, Netherlandwhere maritime industry contribute immensely to their economy.
Understanding Cyberattacks in Maritime Operations
Maritime functions within a complex ecosystem of ports, shipping companies, logistics providers, and regulatory authorities. Over the past two decades, ports have progressively depended on automated information and operational technologies. This digital reliance creates vulnerabilities that, in the case of a hack or incident, might incapacitate economic activities. In July 2024, a software upgrade implemented by cybersecurity firm Crowdstrikeshutdown Windows services globally, resulting in turmoil at airports and interrupting essential infrastructure, including port facilities.Incidents of this nature prompt critical inquiries regarding maritime cybersecurity measures and the potential economic and physical repercussions that may come from a cyber incident. The most common attack include ransomware, phishing, and hacking of critical systems like Automation Identification System(AIS) or terminal operating systems.
The Maritime Sector’s Economic Impact: Insights from Nigeria, USA, Netherlands, and Japan
Maritime is one of Nigeria most critical sector, with its port accounting for over 70% of the region’s trade volume in West Africa. The industry has significantly contributes to Nigeria’s Gross Domestic Product(GDP), facilitating oil exports, which makes up over 90% of the country’s foreign exchange earnings. Surprisingly, the country’s maritime industry is vulnerable to cyber threats due to limited cybersecurity professionals, measures and the usage of legacy systems still in existence. More than 95% of cargo entering the United States is transported via ship and port activities, contributing approximately $5 trillion to the annual economy.The marine industry in Japan is vital to its economy, particularly due to the country’s dependence on maritime transport for over 99% of its international trade and the transportation of products and passengers among its many islands.
The marine sector is fundamental to the Dutch economy, embodying the Netherlands’ extensive nautical legacy and critical role as a European trading center. In 2022, the maritime cluster, which includes shipping, shipbuilding, ports, and maritime services, generated a revenue of €95.2 billion. This activity produced a direct added value of roughly €25.9 billion, with an indirect contribution of €5.2 billion, resulting in a total of €31.1 billion.
Notable Incident of Cyber attacks
The International Maritime Organization (IMO) in 2020, fell victims of cyber attack that has ripple the effect of global maritime operation. In 2023, a major ports in Japan suspend operation due to ransomware attack which believes have emanated from Russia. The Port of Nagoya, responsible for approximately 10% of Japan‘s overall trade volume and managing some automobile exports for corporations such as Toyota, suspended its cargo operations on Tuesday, including the loading and unloading of containers onto trailers, following the incident.These incidents revealed weaknesses and highlighted the economic implications associated with cybersecurity in the maritime sector.
Impact of Cyber-attacks on Nations Economy
Cyberattacks often lead to operational downtime in ports resulting in delays of cargo handling and shipping schedules. In Nigeria, where ports like Apapa and Tin Can Island are already struggle with congestion, cyberattack disruptions could exacerbate inefficiencies, causing financial losses for shipping companies and businesses relying of time delivery of their goods.
Frequently cyber incidents lead to higher insurance premiums for maritime operators, insurers factors in cyber risk when underwriting policies, making costlier for shipping companies to secure comprehensive coverage.In every cyber-attack, its comes with consequences which include reputation damage. Cyber incidents destroy the company image and loss of consumer trust. The affected ports or shipping companies would look less attractive to international shipping lines and customers. This reputational destruction can have long-term economic effects, reducing countries competitiveness on maritime environments.
For example, takes Nigeria as the primary exporter of crude oil whose revenue relies heavily on its maritime sector. Cyber attack that disrupt port operations can lead to massive revenue losses. Delay in oil shipment due to compromised systems directly impact foreign exchange earnings and the broader economy.Recovering from a cyberattack involves substantial financial outlays for systems restoration, data recovery, and implementation of upgraded security measures. For a developing economy like Nigeria, these costs can strain already limited resources.
Why Cybersecurity in Maritime Operations Is Essential
The maritime sector is essential infrastructure; thus, preserving its cybersecurity is vital for safeguarding national interests, including energy exports, trade, and employment. A robust cybersecurity framework and measures enhanced the confidence of international stakeholders and customers in marine operations, hence generating increased commerce and investment. Investing in cybersecurity infrastructure and people development is more economical than the financial repercussions of a successful cyberattack. They mitigate risks, facilitating more efficient operations and financial stability.
Steps Toward Strengthening Cybersecurity Maritime Sector
The government of each country, through its marine administration and safety agency, must adopt effective cybersecurity policies specifically designed for the maritime sector. These rules must conform to international standards, including the International Maritime Organization’s principles on maritime cybersecurity. Training for port operators, shipping industry personnel, and other stakeholders on cybersecurity best practices is essential for capacity building. Competent individuals can recognize and alleviate threats prior to their escalation. Upgrading outdated technology systems, implementing modern cybersecurity solutions, and employing AI systems for threat detection will improve resistance against cyberattacks.
The world is a global village due to technology’s profound interconnectedness of our actions. Collaboration among government, business sector, and international partners is key in mitigating cyberattacks. Exchanging knowledge on cyber threats and implementing a cohesive strategy can enhance defenses across continents and regions.Formulating rapid response teams and contingency plans to ensure operations can swiftly recover following a cyber-attack will reducing economic losses and operational decline.
Conclusion
The economic impact of cyberattacks on maritime operations is a stark reminder of the price of neglecting cybersecurity. As the nation’s aspires to be a viable economy powerhouse of their regions, protecting its maritime sectors and national security from cyber threats must be a top priority. Develop a proactive measure, strong polices and strategic investments in technology will not only safeguard the industry but also bolster nations position in the global maritime landscape. The failure of government to act decisively risks costly disruptions, revenue losses, and reduce competitiveness. A price no economy can afford to pay.
Technology
Cybersecurity as a Business Priority: Experts to Lead Discussion at EyBrids Global Conference
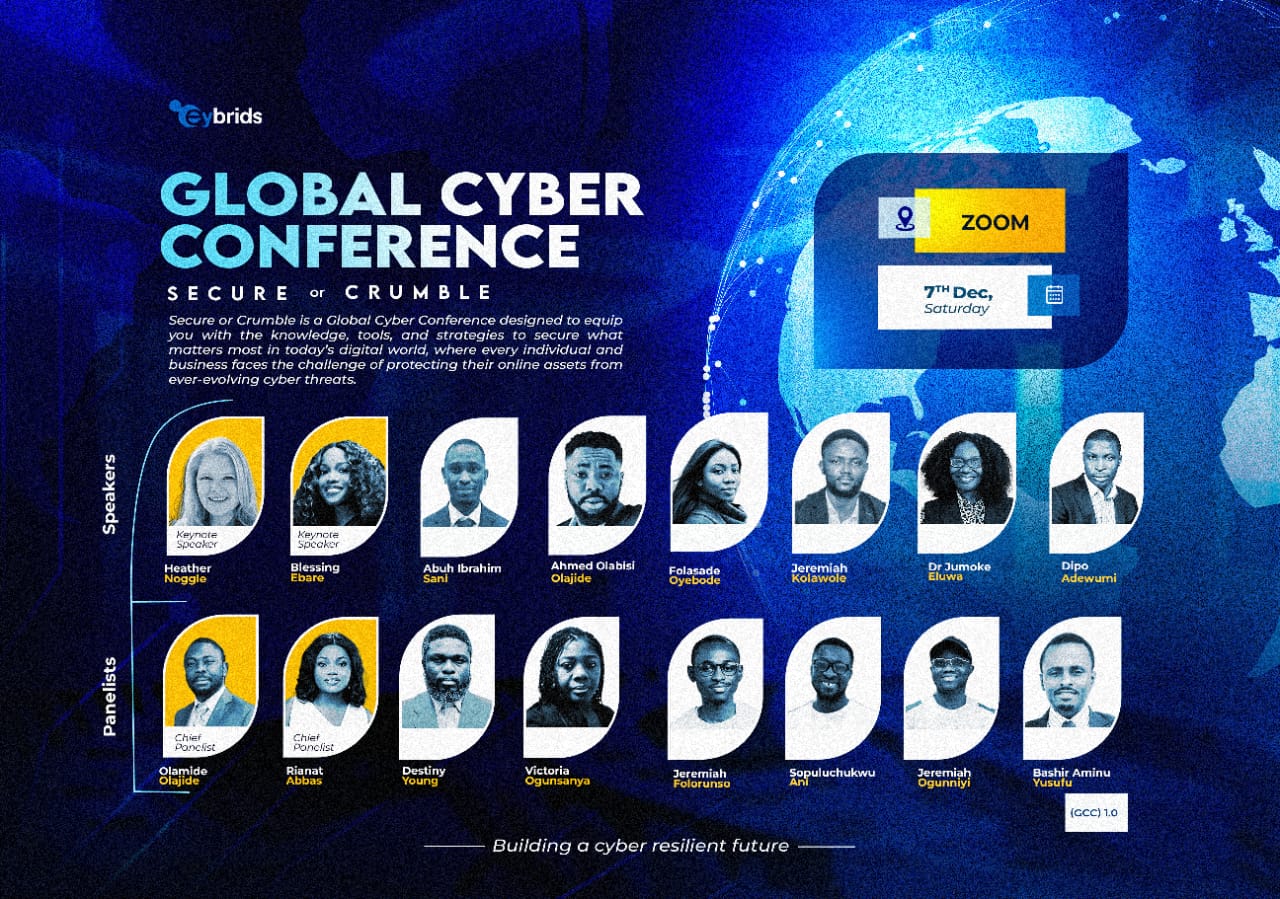
EyBrids, an emerging tech startup recognized for its innovative solutions, has revealed the remarkable lineup of the distinguished speakers and panelists for its upcoming Global Cybersecurity Conference, themed “Secure or Crumble: Building a Cyber Resilient Future”.
As the highly anticipated Global Cybersecurity Conference, organized by EyBrids, draws closer, attention turns to one of the panel sessions, “The Business Case for Robust Cybersecurity.” This session will be led by Rianat Abbas, a seasoned product security analyst, and Victoria Ogunsanya, a professional cybersecurity analyst, who will guide the discussion on how cybersecurity is no longer just a technical consideration but a vital business priority.
In a statement released by the event organizers, Abuh Ibrahim Sani underscored the importance of the session and its leaders. “Cybersecurity has evolved from being a purely technical issue to a key driver of business resilience and growth. With Rianat and Victoria leading this discussion, participants will gain actionable insights on how strategic cybersecurity investments can safeguard operations, protect customer trust, and drive long-term success,” he said.
Rianat Abbas, known for embedding robust security measures throughout the product lifecycle, will bring her expertise to discussions on aligning cybersecurity with product innovation and development. Victoria Ogunsanya, with her focus on proactive threat detection and mitigation, governance and risk management will share strategies for helping businesses stay ahead of emerging risks while maintaining operational stability. Together, they will emphasize the critical role of cross-functional collaboration in transforming cybersecurity from a cost center into a strategic enabler of success.
This session, led by two of the conference’s most dynamic thought leaders, is set to provide attendees with practical strategies and forward-thinking approaches to address the evolving cybersecurity landscape while meeting broader business objectives.
The conference, scheduled for December 7, 2024, at 5 PM GMT via Zoom, will feature an outstanding lineup of speakers and panelists, including Ahmed Olabisi, a renowned cybersecurity expert; Olabode Folasade, a skilled Data Analyst; Dr. Olajumoke Eluwa, a distinguished Cybersecurity Professional; Jeremiah Kolawole, a leading Cybersecurity Professional; Heather Noggle, Executive Director of the Missouri Cybersecurity Center of Excellence; and Blessing Ebare, a seasoned Information Security Professional.
The panelists for the event include Olamide Olajide (Chief Panelist), a seasoned Elasticsearch Data Engineer; Rianat Abbas (Chief Panelist), a Product Security Analyst driving innovation; Destiny Young, a forward-thinking Cybersecurity Engineer; Jeremiah Folorunso, a creative Product (UI/UX) Designer; Sopuluchukwu Ani, a Senior Business Applications Administrator; Jeremiah Ogunniyi, an experienced Backend Developer; Victoria Ogunsanya, a seasoned Cybersecurity Analyst; and Bashir Aminu Yusufu, a Senior System Analyst.
The panelists, alongside other renowned speakers, will lead discussions on topics such as secure system design, cross-functional cybersecurity collaboration, and innovative approaches to mitigating threats. The conference will also feature interactive sessions, enabling participants to connect directly with experts and peers.
“This conference isn’t just about identifying challenges; it’s about equipping attendees with practical tools and knowledge to tackle them head-on,” Abuh stated. “From business leaders to IT professionals and cybersecurity enthusiasts, there’s something here for everyone.”
Technology
EyBrids Unveils Star-Studded Lineup for Global Cybersecurity Conference
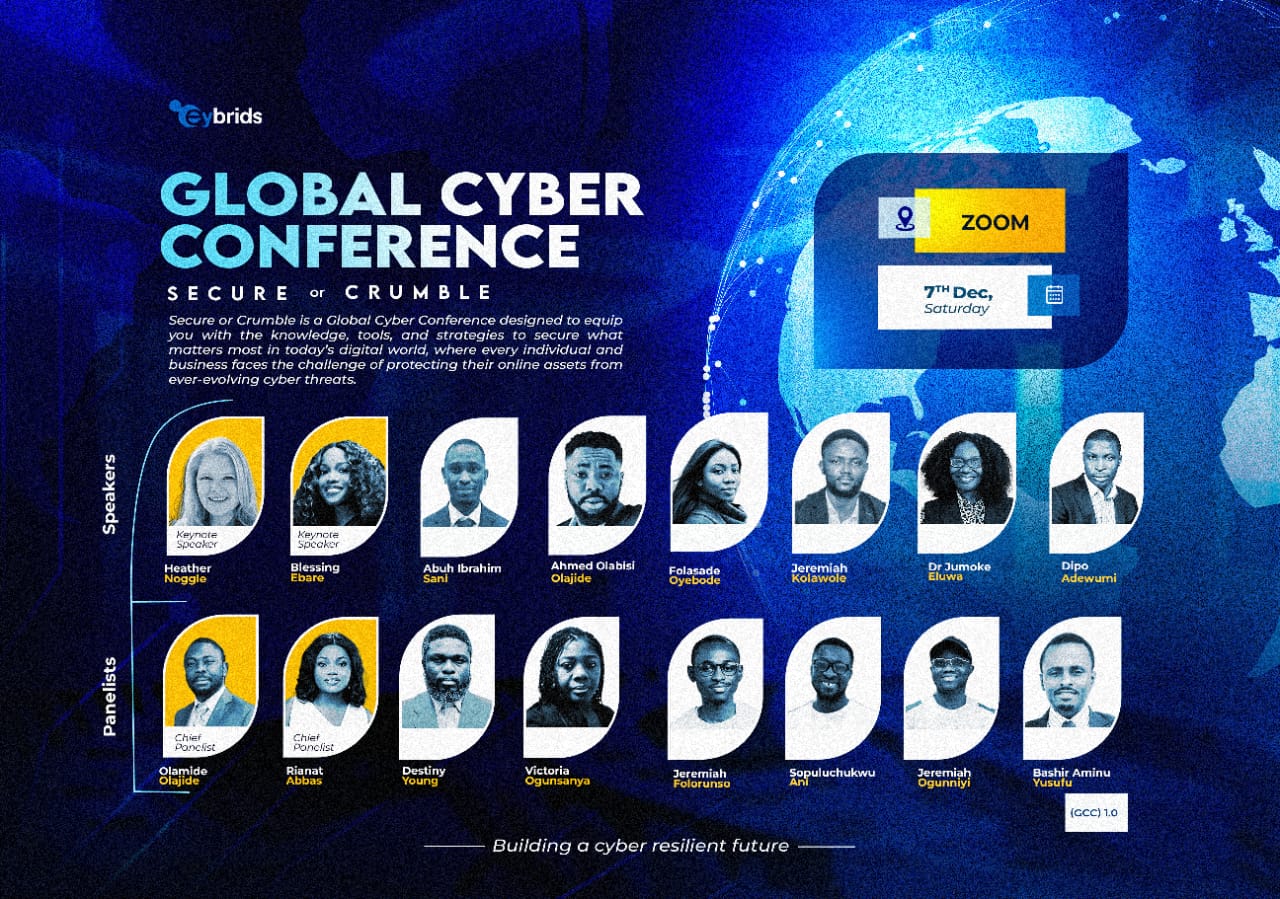
EyBrids, an emerging tech startup recognized for its innovative solutions, has revealed the remarkable lineup of the distinguished speakers and panelists for its upcoming Global Cybersecurity Conference, themed “Secure or Crumble: Building a Cyber Resilient Future”.
In a statement issued by Abuh Ibrahim Sani, one of the event’s organizers, on Wednesday, November 27, 2024, the speakers were described as leading voices in the tech industry, committed to addressing some of the most urgent cybersecurity issues of today.
The conference scheduled for December 7, 2024, at 5 PM GMT via Zoom, promises to foster critical conversations about safeguarding businesses from evolving threats while emphasizing the importance of cross-functional collaboration.
According to Abuh, “Our speakers and panelists represent a wealth of experience across various cybersecurity and tech disciplines, making this conference an unmissable opportunity to learn from some of the best minds in the field.”
He added, “Their collective insights will help attendees understand why organizations must prioritize cybersecurity as a cornerstone for business resilience. Collaboration, innovative strategies, and shared responsibility are key to navigating today’s digital landscape.”
Speakers and Panelists Lineups
The event’s thought-leader panelists will focus on Panel Session 1: “𝘼 𝘾𝙧𝙤𝙨𝙨-𝘿𝙤𝙢𝙖𝙞𝙣 𝙋𝙚𝙧𝙨𝙥𝙚𝙘𝙩𝙞𝙫𝙚 𝙤𝙣 𝘾𝙮𝙗𝙚𝙧𝙨𝙚𝙘𝙪𝙧𝙞𝙩𝙮” and Panel Session 2: “The Business Case for Robust Cybersecurity,” bringing together expertise from diverse fields, including cybersecurity, data engineering, UI/UX design, product analytics, and system architecture. The sessions aim to highlight the importance of cross-domain collaboration in addressing modern cyber threats and aligning security strategies with organizational goals. Speakers at the conference include Ahmed Olabisi Olajide, a renowned cybersecurity expert; Olabode Folasade, a skilled Data Analyst; Dr. Olajumoke Eluwa, a distinguished Cybersecurity Professional; Jeremiah Kolawole, a leading Cybersecurity Professional; Heather Noggle, Executive Director of the Missouri Cybersecurity Center of Excellence; and Blessing Ebare, a seasoned Information Security Professional.
They will be joined by thought-leader panelists such as Olamide Olajide (Chief Panelist), a seasoned Elasticsearch Data Engineer; Rianat Abbas (Chief Panelist), a Product Security Analyst dedicated to embedding security into product life cycles; Destiny Young, a forward-thinking Cybersecurity Engineer specializing in secure network infrastructures; Jeremiah Folorunso, a creative Product (UI/UX) Designer focused on building secure, user-centric interfaces; Sopuluchukwu Ani, a Senior Business Applications Administrator with expertise in safeguarding enterprise systems; Jeremiah Ogunniyi, an experienced Backend Developer skilled in creating resilient system architectures; Victoria Ogunsanya, a proactive Cybersecurity Analyst dedicated to threat detection and mitigation; and Bashir Aminu Yusufu, a Senior System Analyst with expertise in optimizing organizational security. Together, these speakers and panelists will ensure attendees gain practical knowledge, actionable strategies, and fresh perspectives on building cyber resilience and aligning security efforts with business success.
The panelists, alongside other renowned speakers, will lead discussions on topics such as secure system design, cross-functional cybersecurity collaboration, and innovative approaches to mitigating threats. The conference will also feature interactive sessions, enabling participants to connect directly with experts and peers.
“This conference isn’t just about identifying challenges; it’s about equipping attendees with practical tools and knowledge to tackle them head-on,” Abuh stated. “From business leaders to IT professionals and cybersecurity enthusiasts, there’s something here for everyone.”
-
Business3 years ago
Facebook, Instagram Temporarily Allow Posts on Ukraine War Calling for Violence Against Invading Russians or Putin’s Death
-
Headlines3 years ago
Nigeria, Other West African Countries Facing Worst Food Crisis in 10 Years, Aid Groups Say
-
Foreign3 years ago
New York Consulate installs machines for 10-year passport
-
Technology4 months ago
Zero Trust Architecture in a Remote World: Securing the New Normal
-
Entertainment2 years ago
Phyna emerges winner of Big Brother Naija Season 7
-
Business8 months ago
Nigeria Customs modernisation project to check extortion of traders
-
Business10 months ago
We generated N30.2 bn revenue in three months – Kano NCS Comptroller
-
Headlines7 months ago
Philippines’ Vice President Sara Duterte resigns from Cabinet